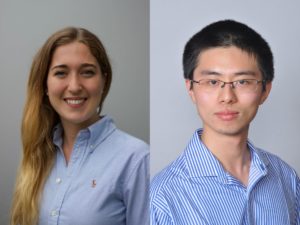
Automatic characterisation of Lipiodol (Guerbet) deposition is feasible, workflow efficient, and improves consistency across patients, argued Sophie Stark (Yale School of Medicine, New Haven, USA) at the Society of Interventional Oncology’s annual meeting (SIO; 7–11 June, Boston, USA). Stark presented an automated quantitative and pattern-based method for the characterisation of Lipiodol deposits on CT scans taken 24 hours after cTACE (conventional transarterial chemoembolization).
“This work paves the way for the development of automated algorithms that predict tumour response from preprocedural MRI and 24 hour CT, and may allow earlier and more personalised therapy”, she enthused at the meeting.
“Lipiodol use in cTACE plays a unique multifunctional role”, Stark explained. “It has drug delivery capabilities with transient microembolic effects, and its radiopacity allows drug delivery visualisation on post-procedural CT scans. Previous studies already show that Lipiodol deposition is correlated with tumour response.”
The investigators within the Yale Interventional Oncology Research Lab, led by Julius Chapiro, set out to see if they could further establish Lipiodol as an early imaging biomarker for the therapeutic efficiency of cTACE using this automated system on 24 hour CT scans. Contextualising this research, Stark informed the SIO audience that liver cancer is the fourth leading cause of cancer-related deaths worldwide, and that cTACE is a guideline approved treatment option for patients with intermediate to advanced stage disease.
They conducted a retrospective study of 42 primary and secondary liver cancer patients, with a total of 65 tumours between them, who underwent cTACE between 2012 and 2018 at the Yale School of Medicine. All patients had a baseline contrast-enhanced MRI scan before undergoing cTACE, then a CT scan 24 hours after the procedure, followed by a second MRI scan 30 days post-procedure. Detailing this image analysis workflow, Stark explained how she and her colleagues volumetrically segmented all of the tumours at all imaging time points. This was followed by an automatic, enhancement-based quantification of viable and necrotic tumour tissue on MRI. Next, the investigators performed an enhanced-based volumetric response assessment using qEASL (Quantitative European Association for the Study of the Liver).
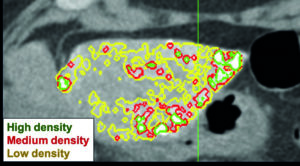
“On the 24 hour CT, which was our focus, we characterised Lipiodol deposition automatically”, Stark continued. The researchers looked at the tumour coverage with Lipiodol, as well as areas of different Lipiodol densities—categorised as low, medium and high—using intensity thresholds from the Hounsfield units scale. The cut-off values were: low density, 87–145 Hounsfield units (HU); mid density, 155–240 HU; high density, 241+ HU. The automated quantification method was developed by one of Stark’s co-workers, Clinton Wang, who is currently a graduate student at the MIT in Boston, USA, and was previously involved with research at Yale. Based on these density values, the developed algorithm creates a colour coded overlay for the CT image of the liver, where low density areas were shaded green, mid density areas shaded yellow, and high density areas shaded red (see Figure 1).
Stark continued: “Specifically, we looked at homogenous deposition of Lipiodol, sparsity, rim deposition of Lipiodol, and peripheral deposition. The homogenous and sparsity deposition of Lipiodol could be determined through volumetric analyses of the whole tumour, whereas for the rim and peripheral Lipiodol deposition, a more complex approach was required.” Expanding on these complexities, she said: “First, we calculated the volume of the tumour, and then created a sphere with exactly the same volume. Then we used operations such as morphological erosion and dilation to separate the core and the periphery of the tumour.”
Lipiodol coverage in viable areas was significantly higher than that of necrotic areas, the investigators found through comparing contrast-enhanced MRI scans. There was approximately 8.2% more Lipiodol coverage in viable areas than necrotic zones, averaged throughout all tumours. “We also looked at several subgroups, and the analysis was consistent throughout”, Stark said.
Looking at Lipiodol density distribution, the fraction of low density deposition was significantly higher in necrotic areas compared with viable areas, whereas the fraction of high density Lipiodol was significantly higher in viable compared to necrotic areas. There was no significant difference for mid density Lipiodol. Also, this analysis was consistent throughout several subgroups, according to Stark.
The investigators reported a stratification of tumour response by Lipiodol density. Areas that deposited Lipiodol became necrotic at a higher rate, as seen on follow up MRI, than areas without Lipiodol (p=0.0475). Higher concentrations of Lipiodol enhanced this effect with increasing Lipiodol densities, there was an increasing percentage of necrotic tissue.
When deposited peripherally, Lipiodol is associated with an improved tumour Homogenous, non-sparse, and rim deposition were all associated with accelerating the slowing of tumour volume growth. In Stark’s words: “Homogenous deposition, non-sparse deposition, and rim deposition within sparse tumours showed an increased decrease of enhancing tumour volume on follow up MRI”.
This led Stark and colleagues to conclude that the automated characterisation of Lipiodol “can be easily incorporated into a standardised framework for cTACE management”. Stark summarised: “Our approach improves the consistency for patients because it leverages the standardised Hounsfield units scale. We show that tumour enhancement on contrast-enhanced MRI can make predictions not only about the presence, but also about the density, of Lipiodol deposition, which is strongly correlated to tumour response to cTACE. Also patterns, including homogenous, non-sparse, rim and peripheral deposition of Lipiodol were positive predictors of tumour response. We think this work paves the way for the development of fully automated algorithms that can predict tumour response from pre-procedural MRI and 24 hour post-procedural CT, and may allow earlier and more personalised therapy to our liver cancer patients”.