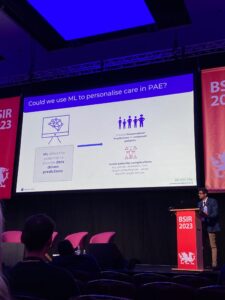
Presenting on machine learning (ML) to predict responders following prostatic artery embolization (PAE) at the British Society of Interventional Radiology (BSIR; 8–10 November 2023, Newport, UK) annual meeting, Ganesh Vigneswaran (University of Southampton, Southampton, UK) sets out his case for the use of ML to “personalise care in PAE, to empower our patients to make better informed decisions and avoid complications”.
Looking first at PAE itself, Vigneswaran averred that therapeutic success is “not the same for everyone”, however bolstered by ML, there is potential to predict who may benefit most from this treatment. “ML is a branch of artificial intelligence,” he explained, “it can find complex patterns in data and works by creating a series of dependant variables or predictors linked with an outcome to build an algorithm that enable it to learn the rules of the game to predict future outcomes with new data”.
To set about proving this hypothesis, Vigneswaran told BSIR delegates, him and his team reviewed data from the UK-ROPE multicentre study which pulled data from 17 UK centres between 2014–2016 including around 216 PAE patients. Inputting urodynamic, radiological and clinical predictor variables, Vigneswaran stated they had over 40 different predictors however there were “significant missing data” which led the investigators to restrict the subanalysis to 6–7 variables to predict international prostate symptom score (IPSS) at one year which showed some “significance”.
“But should we just stop there?” Vigneswaran asked the audience, musing if we can learn more through “analysing our errors”, or if, through “domain expertise” physicians can “move beyond just aiming for an accurate model and actually analyse why”.
Reviewing their data on IPSS reported and predicted outcomes from baseline to one year, Vigneswaran reported some “discordance” between the two, which he believes shows an “underlying role of psychology in PAE outcomes”. He iterates however that “the more data the better”—in order to “increase the power of prediction”, more predictive variables are needed.
Using the example of prostate volume and maximum flow rate (Qmax) at one year in this dataset, the speaker outlined that additional predictors including baseline volume, Qmax, residual volume, Abrams Griffiths number, and IPSS, which resulted in an increase in regression values, notably an increase of 0.05 to 0.27 in Qmax.
Summarising his discussion, he stated that there is predictive value within the clinical radiological and urological variables and avers that more data should be collected. “Machine learning has shown promise in the ability to predict PAE outcomes and our research has shown a clear signal from preprocedural variables”. The speaker went on to note that more data are needed, adding that this will “improve our performance and be used to counsel and guide individual patient decisions in urology and interventional radiology clinic”.